What is generative AI?
Generative AI is a type of artificial intelligence that can create new things, like text, pictures, music, or computer code. It works by learning patterns from a lot of data. Instead of following set rules, generative AI studies large amounts of information and then create something new based on what it has learned. It is, therefore, useful for creative work, like making art or writing content.
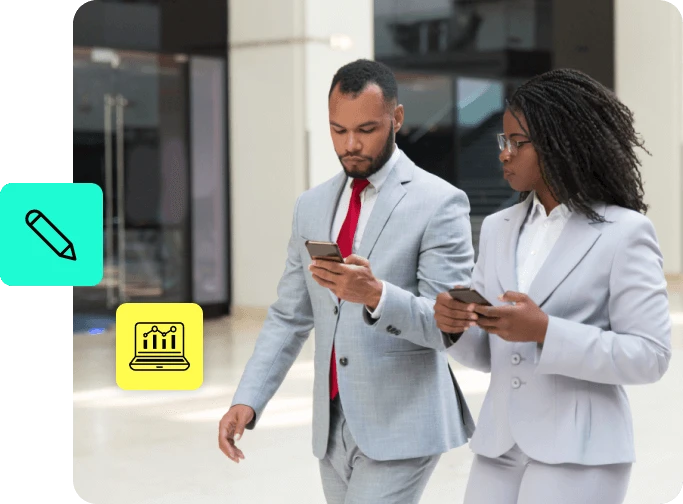
Table of contents
To work well, generative AI needs to be trained. For example, if it’s meant to create pictures, it’s trained on many photos to learn what different objects look like. Once trained, it can make its own pictures that look real but are unique. The same process is used for text, where generative AI can write sentences that make sense by following language patterns it has learned.
Generative AI is used in many areas. Indeed, 65% of McKinsey survey respondents reported their organizations regularly use generative AI. Businesses use it to write ads or help with customer service, and the entertainment industry uses it to create music, design games, and make visuals.
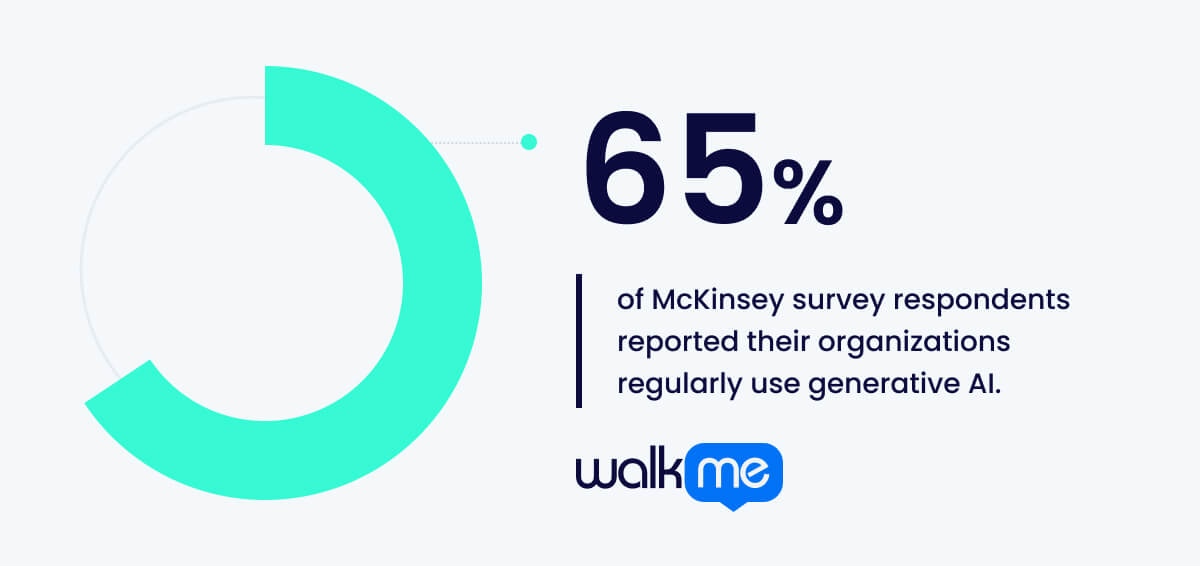
Although it can be helpful and creative, generative AI can also be misused, like creating fake information. So, it’s important to use it carefully and responsibly.
The generative AI market value almost doubled between 2022 and 2023, growing to 45 billion US dollars. Furthermore, the annual growth of 20 billion US dollars annually is projected to continue until 2030.
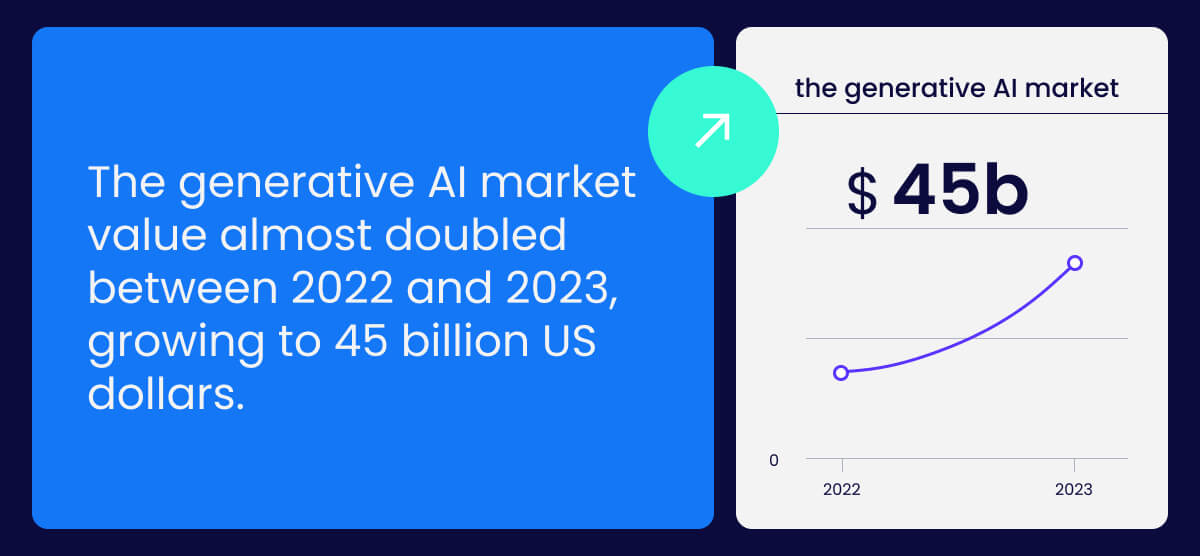
What are the key components of generative AI?
Understanding the parts of generative AI is important for understanding how it creates new things and what makes it work well. Each part helps the AI learn from information, create original ideas, and respond to users’ needs. Together, these parts ensure that the AI can produce interesting and useful results. Let’s look at the key components that help generative AI work.
Data
Generative AI needs a lot of information to learn from. The better and more different the information is, the better the AI can create new things.
Algorithms
Algorithms help the AI use the information. Smart tools, like Generative Adversarial Networks (GANs) and Variational Autoencoders (VAEs), help the AI make new content.
Training
Generative AI needs training to learn from the information. While training, the AI looks at the data to see how different pieces fit together and how to make new ones.
Inference
After training, the AI can make new things based on what it learned. It uses the patterns it found to create sentences, pictures, or music.
Evaluation
It is important to check if the AI’s creations are good. The process involves looking at what the AI made to see if it makes sense and is original. Feedback helps the AI improve.
User interaction
Many generative AI systems let people talk to them. People can give ideas or ask for certain things, helping the AI make what they want.
Ethics and governance
As generative AI improves, it’s important to consider how it is used. Issues like fairness and safety can help ensure that generative AI is used effectively.
Generative AI vs. traditional AI vs. predictive AI
Generative AI, traditional AI, and predictive AI are three types of artificial intelligence that work in different ways.
- Generative AI makes new things, like pictures or text, that look real.
- Traditional AI follows rules to do specific tasks, like answering questions or recognizing faces.
- Predictive AI looks at past patterns to make guesses about the future.
Let’s look at how these three types of AI are different.
Generative AI | Traditional AI | Predictive AI | |
Definition | Creates new things, like pictures, text, or music. It learns patterns from lots of data and can make something that looks real, even though it’s computer-made. | Follows set rules to do specific tasks, like spotting faces in photos or answering basic questions. It works well when the task is straightforward and doesn’t change much. | Guesses what might happen in the future by looking at patterns in past data. It’s used to make smart predictions, like what a customer might buy next. |
Focus | Making new content that looks, sounds, or reads like it was made by a human. | Doing specific tasks based on set rules or instructions without changing. | Figuring out what could happen next by spotting patterns in data. |
Techniques | Uses special models to create new things, like pictures or stories. | Uses rules and simple models to follow instructions and get tasks done. | Uses math and patterns to guess what could happen in the future. |
Goals | Create things like art, stories, or human-like conversations. | Do specific, repeatable tasks accurately, like spotting fraud or answering questions. | Help plan for the future by predicting what could happen. |
Examples of use | Creating realistic pictures, writing stories, or chatting like a real person (e.g., ChatGPT). | Answering customer questions with chatbots or spotting faces in photos. | Guessing how many people will buy something or if someone will pay back a loan. |
Use cases for generative AI
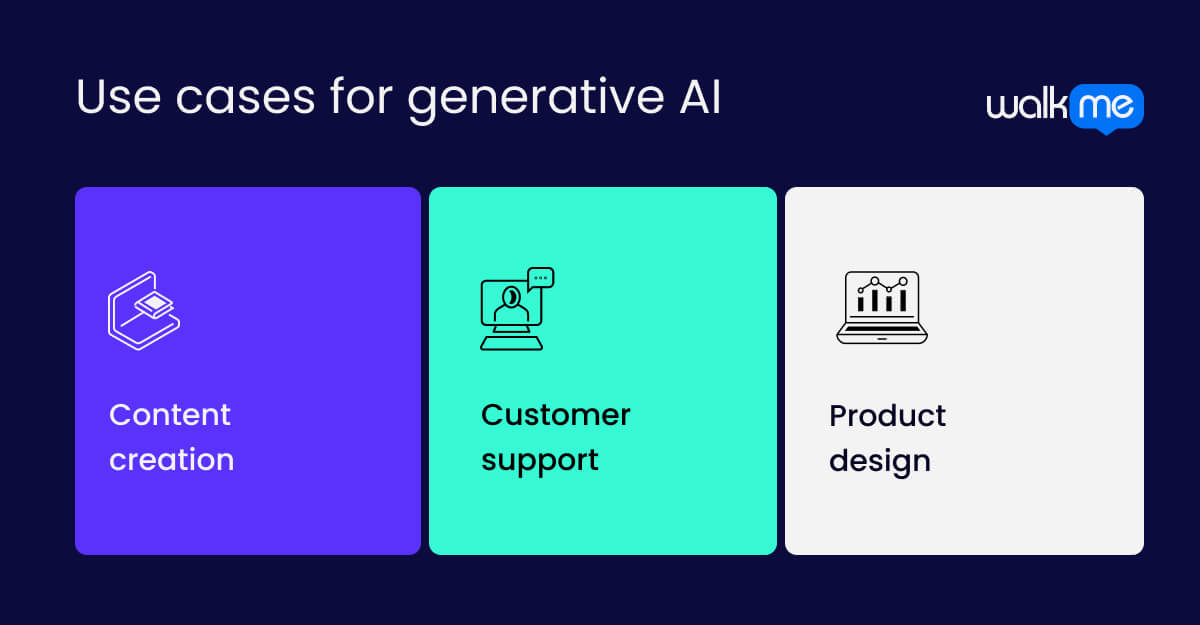
Generative AI is useful in many business situations.
It helps with creating content and improving customer support, making work easier and quicker for companies.
It can be hard to see how generative AI is used in real life, so here are three examples that show how it helps in different business areas.
Content creation
Generative AI helps businesses create content quickly and easily. The system can write articles, blogs, or social media posts on given topics.
It can also suggest catchy titles and even create images to accompany the text. Thus, the marketing team can produce fresh content faster without spending a lot of time writing or designing. As a result, they can focus on other important work and keep their audience interested.
Customer support
Technology can improve customer support by using smart chatbots. These chatbots can understand what customers ask and give helpful answers automatically.
When a customer needs help, the chatbot uses AI to create friendly and clear responses. It speeds up the time it takes for customers to get answers, so they don’t have to wait long. With AI taking care of common questions, human agents can focus on more complicated problems, leading to better support overall.
Product design
Those in product design can use generative AI to help with new ideas. The system looks at existing products and customer feedback to create designs that fit what users want.
It can generate different options for colors, shapes, and features, allowing designers to explore many choices quickly. The setup speeds up the design process and helps companies create products that customers will like. In the end, businesses can launch new and exciting products faster and stay ahead of their competitors.
What are the advantages of generative AI?
Generative AI has many benefits for businesses. This technology helps companies create new content and ideas quickly and easily. It also saves time and lets workers focus on important tasks that require human creativity.
Let’s look at the different ways generative AI can help businesses succeed.
Increases creativity
Generative AI helps create new ideas and designs that people might not think of on their own. By looking at a lot of information, it finds special patterns that spark fresh concepts. The ability to come up with new ideas makes team meetings more fun and productive. With the unique ideas generated, teams can make more interesting and original content for their projects.
Reduces costs
Using AI can save businesses a lot of money. By quickly doing simple tasks, it cuts down on the need for extra workers in those areas. The money saved can then be used for more important projects that help the company grow. Over time, the savings from using AI can provide the resources needed for bigger initiatives.
Improves personalization
With the right prompts, AI can create content specially made for each user based on what they like. Marketing messages can be changed to better connect with each customer, making them feel valued. Providing a personal touch makes customers more interested and builds a stronger bond with the brand. As a result, businesses enjoy happier customers who keep coming back.
Speeds up processes
Generative AI can write reports or generate marketing content much faster than humans. This speed helps businesses respond quickly to changes and customer questions, keeping them ahead of the competition. By saving time on simple tasks, teams can focus on more important work that requires creativity and problem-solving. Overall, this leads to better efficiency and quicker decision-making in the company.
Enhances decision-making
AI makes analyzing large data sets easy. The tool can provide helpful insights for making smart business choices. By spotting trends, risks, and opportunities that may not be easy to see, organizations can plan better. More accurate information leads to more effective strategies and good results. As a result, companies become more flexible and ready for new challenges.
Boosts productivity
By automating simple tasks, employees can focus on more important work that needs creativity and thinking. The change allows teams to get more done in less time, increasing overall productivity. Workers can spend their energy on valuable activities instead of routine tasks. Such a shift leads to a more engaged workforce and higher output for the company.
Supports collaboration
AI tools help team members share ideas more easily. By giving suggestions and insights, these tools encourage everyone to build on each other’s thoughts. Such teamwork creates a culture of innovation, where different views are explored and valued. With AI as a helpful partner, teams can boost their creativity and problem-solving skills.
Provides scalability
Thanks to AI, companies can take on more work without needing to hire more people. As businesses grow and start new projects, AI can manage repetitive tasks while maintaining high quality. The ability to scale allows organizations to grow while keeping costs down. Therefore, companies can focus on development and new ideas without facing typical limits.
What are the challenges of generative AI?
Now let’s look at the challenges of using generative AI.
While this technology has the potential to create amazing content and solutions, it also brings some difficulties that businesses must face.
Understanding these challenges is key to making the most out of generative AI while avoiding potential problems.
Let’s dive into the main challenges that come with adopting and using generative AI effectively.
Data quality
Generative AI needs a lot of good data to work well. If the data is accurate and needs to include important parts, the results can be good. Cleaning and organizing data can take a lot of time and effort. Without high-quality data, the AI might not create the right content or solutions.
Ethical concerns
There are worries about how generative AI can be used. It can create fake news or misleading information, which can harm people or businesses. Companies must think about how to use this technology responsibly. Setting up clear rules and guidelines is important to prevent misuse and protect their reputation.
Cost of implementation
Using generative AI can be expensive. Businesses may need to buy special tools and hire skilled workers to use them. The costs of training and maintaining the system can add up quickly. Companies must plan their budget carefully to make sure they can afford to use this technology without overspending.
Understanding results
Sometimes, generative AI’s outputs can be hard to understand. It can create content that seems good but may not make sense or be useful. Workers need to spend time reviewing and checking the results. Doing extra work can slow down the process and reduce the overall efficiency of using AI.
How can DAPs help with generative AI?
Digital adoption platforms (DAPs) are very helpful for using generative AI in businesses. When companies use a DAP, it makes it easier for them to work with generative AI tools. The platform provides support and resources that help people create good content and solutions quickly.
Let’s look at how DAPs make it easier to use generative AI and help businesses do better.
Streamlining workflows
DAPs make work easier by bringing generative AI tools directly into daily tasks. Users can find and use AI features without having to switch between different apps. Simplifying the process helps everyone create and use AI-generated content more effectively. As a result, teams can focus on their jobs instead of getting stuck in complicated steps.
Providing guidance
Clear guidance on how to use generative AI tools is a key benefit of DAPs. Step-by-step instructions and helpful tips help users know how to create content or solutions successfully. Such support builds user confidence and encourages exploration of all the great things generative AI can do. Consequently, teams can produce better work with less frustration.
Monitoring performance
Another important role of DAPs is to check how well generative AI is working. They keep track of user engagement and the effectiveness of AI-generated content, helping teams see what works best. Useful information about tool usage allows DAPs to suggest ways to improve, leading to better results from the AI tools over time.
Encouraging collaboration
DAPs greatly enhance team collaboration when using generative AI. They create shared spaces where users can work together on projects and share AI-generated content. Boosting creativity lets users learn from one another and encourages innovative ideas. By bringing teams together, DAPs help everyone maximize the benefits of generative AI.