What is AI optimization?
AI optimization means improving how artificial intelligence (AI) works so it can do tasks better and faster. The process involves changing the AI’s “rules” to make it smarter and more accurate, using less power and memory. Optimizing AI helps it work well in areas like helping customers, managing money, or even in healthcare.
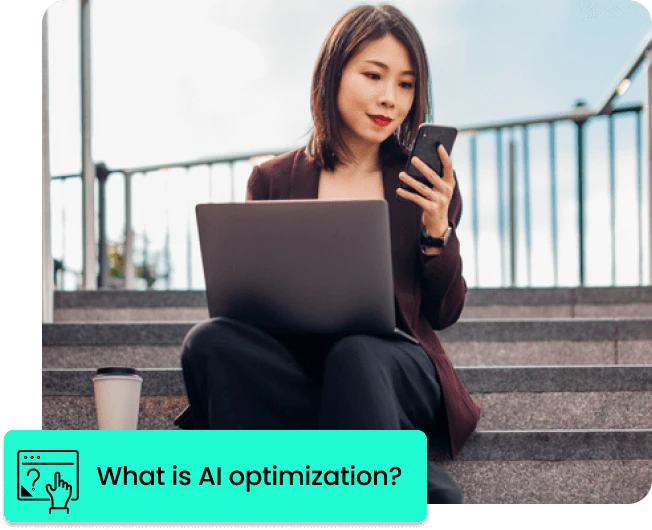
To start, people look at what needs fixing or improving in AI. For example, if a customer service AI is slow at answering, making changes might speed it up by adjusting how it processes information. Some approaches include training the AI with new data or changing the way it handles questions. The goal is for the AI to do its job as well as possible.
By optimizing AI, businesses can save time and money, making it work more efficiently. Experts often need to test and adjust it to keep it running smoothly. As a result, AI can give better service, help people work faster, and make good use of resources.
What are the key steps involved in AI optimization?
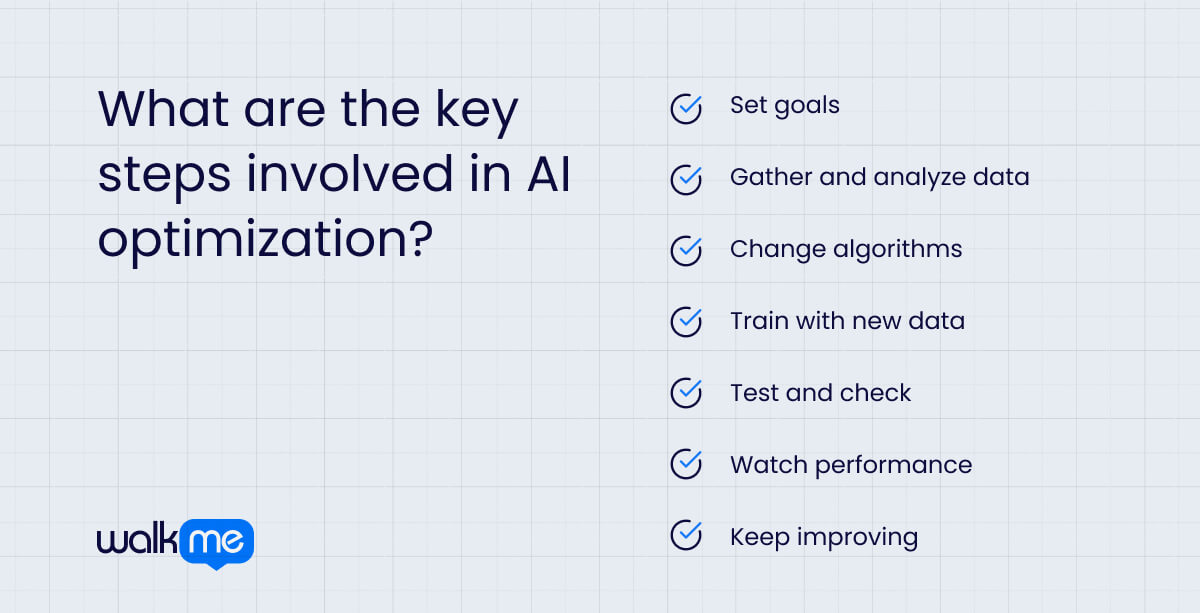
Understanding the key steps in AI optimization is important for making AI systems work better and faster. Each step, from setting clear goals to training the AI and checking its performance, helps turn AI into a useful tool that can help a business.
Let’s look at the main steps in optimizing AI and see how they work together to make it more effective and ensure it gives the best results.
Set goals
First, it’s important to decide what needs improvement. Improving the AI could mean making it more accurate, faster, or using less power.
Gather and analyze data
Collecting the right data is crucial for understanding how well the AI is doing. Analyzing the data helps find areas where it can get better.
Change algorithms
Tweaking the rules that guide the AI is essential. Adjusting settings, choosing different models, or using new methods can boost performance.
Train with new data
Training the AI with fresh or extra data helps it learn better. This can make it more accurate and improve its output.
Test and check
After making changes, it is important to test the AI to see if the adjustments worked. It is also crucial to ensure the AI meets the goals set earlier.
Watch performance
It is key to monitor how the AI works over time. Regular checks help ensure that it stays effective and can be improved further.
Keep improving
Optimizing AI is a continuous job. Based on what is learned from monitoring, more changes can be made to keep making the AI better.
AI optimization vs. machine learning vs. generative engine optimisation
AI optimization, machine learning, and generative engine optimization are types of artificial intelligence, but they do different things.
Let’s explore the differences between these three ideas.
Use cases for AI optimization
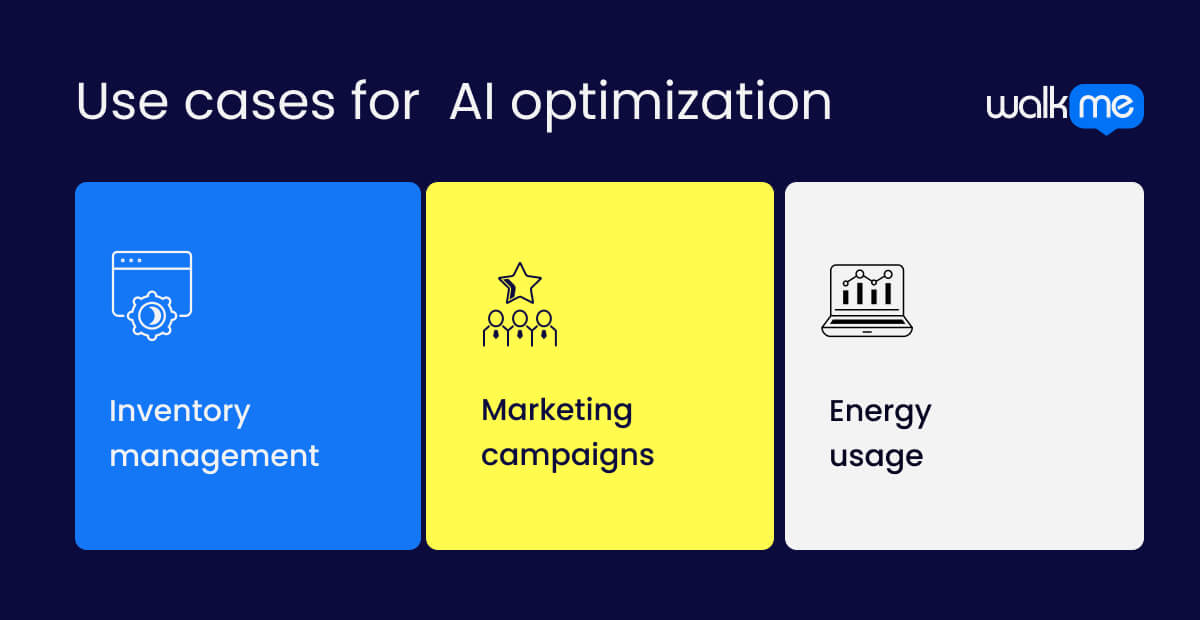
AI optimization is important for many businesses.
It helps in different areas, like keeping track of inventory and making marketing better.
For example, Walmart uses AI to create a more efficient supply chain and Siemens uses predictive maintenance in manufacturing.
Seeing how AI optimization works in real-life situations can be tricky, so here are three examples that show how it can help businesses in different ways.
Inventory management
AI allows businesses to better manage their inventory. The system collects data about what is being sold and how much stock is always present.
It uses this information to predict how much stock will be needed in the future, looking at trends in sales and popular items. By analyzing these patterns, businesses can ensure they do not run out of popular items or have too many items that don’t sell well.
Companies save money while always having the right products available for customers when they want them. Good inventory management not only keeps customers happy but also helps the business grow and succeed.
Marketing campaigns
AI improves marketing campaigns by studying customer data closely. The system looks at what customers have bought in the past and what they like to do.
Then, it suggests the best ads to show to different groups of people based on their interests and needs. By reaching the right customers with the right messages at the right time, businesses can sell more products and get better results from their marketing efforts.
Such an approach helps make marketing much more successful and can create stronger connections with customers. Over time, businesses can build better relationships with their customers, making them feel valued and appreciated.
Energy usage
AI helps businesses use less energy in their daily work. The system continuously checks how energy is used throughout the company to find where energy is being wasted.
After analyzing this data, the system provides helpful tips on how to save energy, such as adjusting heating or cooling settings to more efficient levels. By using energy more wisely, companies can lower their bills, which saves them money and helps protect the environment.
Smart energy use improves business operations and benefits the planet by reducing waste and pollution.
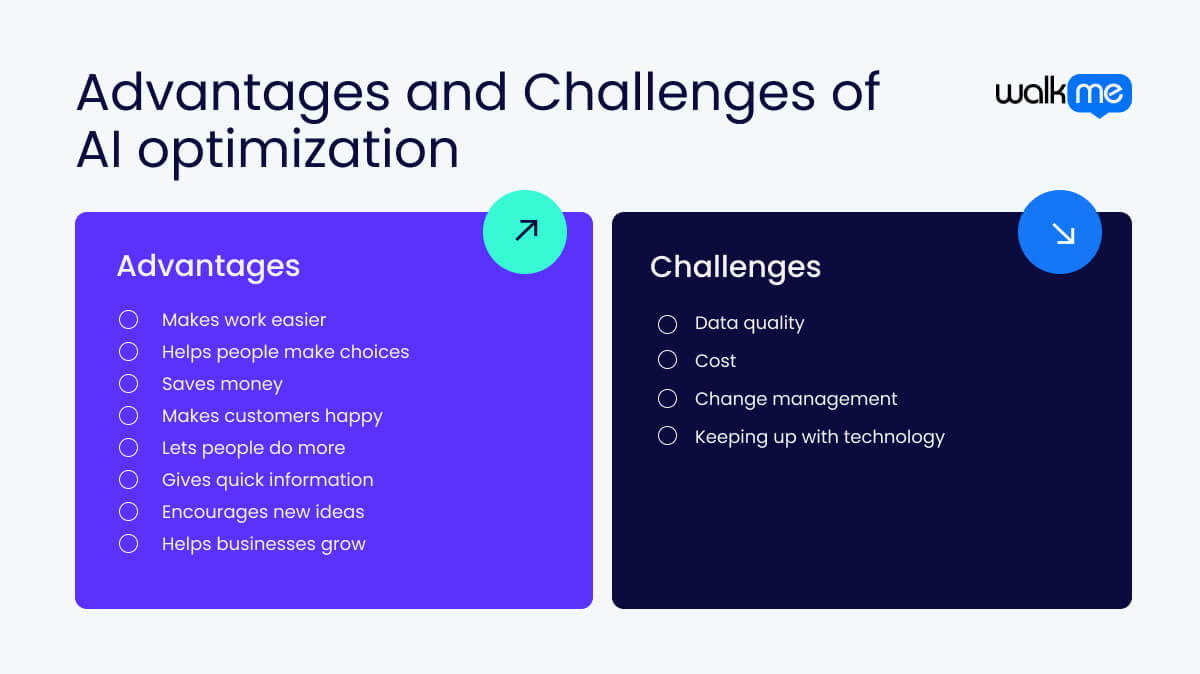
What are the advantages of AI optimization?
AI optimization has many advantages for businesses that want to improve how they work. Using smart technology helps companies do their tasks faster and make better choices. The setup can lead to happier workers, satisfied customers, and more money for the business.
Let’s look at the different benefits of using AI optimization in various business situations.
Makes work easier
AI optimization helps businesses do their tasks faster and smoother than before. By picking the best ways to get things done, it reduces waiting times and helps everyone work better together. Workers can finish their jobs more quickly, allowing them to focus on what really matters to the company and its goals. Overall, this makes the entire operation run more smoothly, leading to better results and a happier workplace.
Helps people make choices
The technology looks at a lot of information to find important details that people might miss. Its ability to analyze large amounts of data helps bosses make better choices for their company. With clear ideas and useful insights, they can plan what to do next more easily and confidently. As a result, the company can adapt to changes more quickly, allowing it to thrive and grow in a smart way.
Saves money
Using AI can help businesses spend less money by making their work more efficient and effective. By streamlining processes and improving performance, AI cuts down on wasted time and resources, leading to significant cost savings. The money saved can then be redirected toward other important initiatives that can help the business grow. In the end, this not only leads to increased profits but also strengthens the overall financial health of the company.
Makes customers happy
Businesses can serve their customers better than ever before. By improving how they manage operations and respond to inquiries, companies can answer customer needs much faster and provide top-notch service. When customers receive quick and helpful responses, they are happier and have a better experience with the brand. A satisfied customer is more likely to return for future purchases and recommend the business to their friends, boosting the company’s reputation.
Lets people do more
Workers finish their tasks more quickly, as AI takes care of boring, repetitive jobs that can slow them down. When machines handle these routine tasks, people can devote their time and skills to more important and creative work. The shift in focus means that more work gets done in less time, making the entire team more productive. Achieving more helps the business grow and meet its goals more effectively.
Gives quick information
By analyzing data instantly, AI provides businesses with important information when they need it most. Such quick access to data helps companies make fast changes and adjustments in response to customer needs or market trends. By staying updated and aware of what’s happening, businesses can adapt their strategies and improve their services. Fast information leads to smarter choices and helps the company stay ahead of the competition.
Encourages new ideas
As AI takes over regular tasks, people can think of new and creative ideas for their business. With machines handling routine work, employees can spend more time brainstorming and coming up with innovative solutions. This freedom can lead to exciting new products or services that capture customers’ interest and enhance their experience. By fostering creativity, businesses can stand out in the market and better meet their customers’ evolving needs.
Helps businesses grow
As a business gets bigger, AI can easily keep up with increased demands without needing extra workers. It can handle more work and tasks without any problems, making it easier for companies to expand confidently. Such an ability to grow effectively is very important for long-term success and allows businesses to take on new challenges without losing quality. Embracing AI helps companies thrive in a competitive landscape while ensuring they maintain high standards of service.
What are the challenges of AI optimization?
Now let’s look at the challenges of AI optimization.
As businesses try to use AI to improve their work, they face several important problems.
These challenges can make it hard to get the most out of AI and achieve the desired results.
Understanding these issues is key to successfully adopting AI and making it work effectively for the company.
Data quality
For AI to work well, it needs good data. If the data is wrong, old, or missing important parts, the results can be poor. Making sure the data is clean and accurate can take a lot of time and effort. Without good data, AI can’t help the business as much as it should.
Cost
Using AI can be expensive. Companies need to pay for tools, software, and sometimes special workers with the right skills. These costs can add up quickly, especially for smaller businesses. It’s important for companies to think about whether the benefits of using AI will be worth the money spent.
Change management
When a business wants to use AI, it often means big changes for how people work. Some workers might feel scared or unsure about using new technology. Helping everyone get used to these changes can take time and support. If workers don’t accept the new ways of working, it can slow down progress.
Keeping up with technology
AI is always changing and getting better. Companies need to keep up with these changes to stay competitive. They must regularly update their tools and worker training. It can be hard to stay on top of everything, and some businesses may fall behind if they don’t adapt quickly.
How can DAPs help with AI optimization?
Digital adoption platforms (DAPs) are helpful for making AI work better. When businesses use a DAP, they can improve how their AI systems perform. These platforms provide important tools that show users how to change AI settings and processes, helping the technology work at its best.
Let’s look at how DAPs help companies get the most out of their AI and make their work more efficient.
Guided learning
DAPs provide guided learning to help users understand how to optimize AI. They include step-by-step lessons that show how to adjust AI settings and improve its performance. Users can follow these lessons at their own speed, making it easier to learn how to make AI smarter and faster. Such support helps everyone feel more confident in using AI to enhance their work.
Real-time assistance
Users receive real-time help while optimizing AI systems. DAPs offer tips and hints right when needed, which reduces the time spent searching for solutions. Immediate support makes the process smoother and helps users quickly make necessary adjustments to improve AI. Fast assistance leads to fewer mistakes and better outcomes.
Easy integration
They make it simple to connect AI optimization tools with existing systems. DAPs help link everything together so users can easily see and manage all their data. Easy integration means businesses spend less time setting things up and more time focusing on improving AI performance. When everything works well together, companies operate more efficiently.
Performance tracking
DAPs help monitor how well AI optimization is working. They provide clear reports and insights that show users which changes are effective and which need more work. With this information, users can make smart decisions to enhance AI and ensure it provides the best service possible. Good tracking leads to improved results and helps businesses succeed.
Frequently asked questions
AI optimization identifies usage patterns, predicts demand, and automates resource allocation, leading to significant reductions in cloud expenditures and improved operational efficiency.
Ongoing monitoring ensures AI systems adapt to changing data and environments, maintaining performance and preventing model drift, which is crucial for sustained effectiveness.
Enterprises often encounter data quality issues, integration complexities, and a shortage of skilled personnel, which can impede effective AI optimization.
By analyzing user behavior and preferences, AI optimization enables personalized content delivery and responsive interfaces, enhancing user engagement and satisfaction.
AI optimization forecasts demand, identifies potential disruptions, and streamlines logistics, resulting in more resilient and efficient supply chain operations.